Research
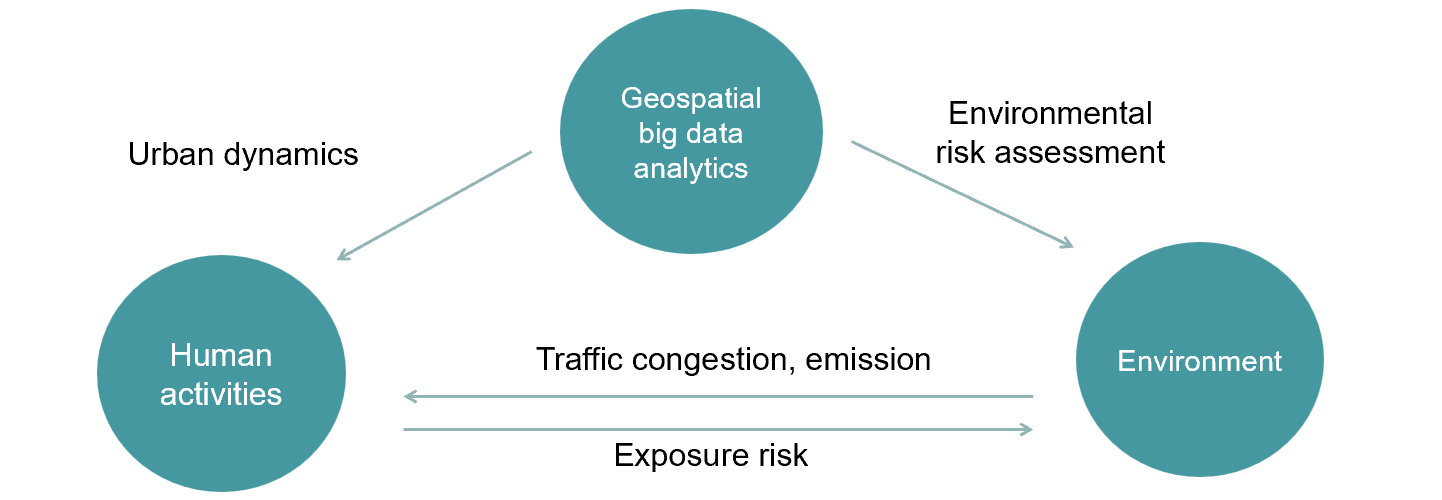
Based on human/vehicle trajectory data, my research develops analysis methods that consider spatial constraints and spatial heterogeneity to comprehensively analyze and understand human dynamics in a fine-grained level. By incorporating these factors into my research, I aim to gain insights into urban dynamics and how human behavior and movement patterns are influenced by the surrounding spatial context.
Kan, Z., Liu, D., Yang, X. and Lee, J., 2024. Measuring exposure and contribution of different types of activity travels to traffic congestion using GPS trajectory data. Journal of Transport Geography, 117, p.103896.
Kan, Z., Kwan, M.P. and Tang, L., 2022. Ripley’s K-function for network-constrained flow data. Geographical Analysis. 54(4), pp.769-788.
Kan, Z., Tang, L., Kwan, M.P. et al., 2019. Traffic congestion analysis at the turn level using Taxis’ GPS trajectory data. Computers, Environment and Urban Systems, 74, pp.229-243.
Tang, L., Kan, Z.*, Zhang, X., et al., 2016. A network kernel density estimation for linear features in space–time analysis of big trace data. International Journal of Geographical Information Science, 30(9), pp.1717-1737.
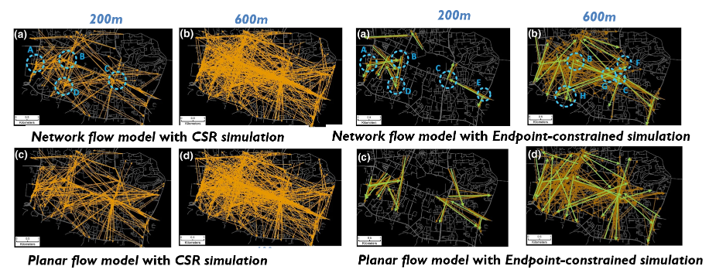
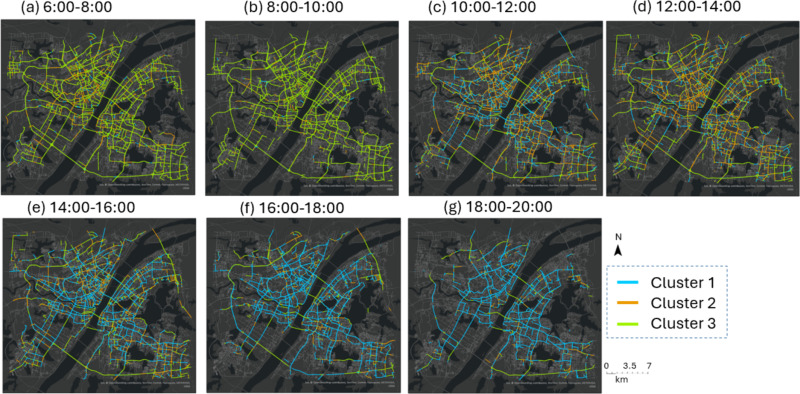
Using data collected from mobile sensing technologies such as GPS, air pollution sensors, and noise sensors, my research focuses on investigating individual mobility patterns and their relationship with environmental exposures. I explore the complex interactions between individual mobility, environmental factors, and health outcomes. By analyzing these relationships, I strive to uncover insights into how environmental exposures during mobility impact human health and well-being, which may enable evidence-based interventions and policies to promote healthier and more sustainable living environments.
Kan, Z., Kwan, M.P., Cai, J., Liu, Y. and Liu, D., 2023. Nonstationary relationships among individuals’ concurrent exposures to noise, air pollution and greenspace: A mobility-based study using GPS and mobile sensing data. Health & Place, 83, p.103115.
Kan, Z., Kwan, M.P., Huang, J., Cai, J. and Liu, D., 2023. A Spatial Network-Based Assessment of Individual Exposure to COVID-19. Annals of the American Association of Geographers, pp.1-11.
Kan, Z., Kwan, M.P., Liu, D., Tang, L., Chen, Y. and Fang, M., 2022. Assessing individual activity-related exposures to traffic congestion using GPS trajectory data. Journal of transport geography, 98, p.103240.
Kan, Z., Kwan, M.P., Ng, M.K. and Tieben, H., 2022. The impacts of housing characteristics and built-environment features on mental health. International Journal of Environmental Research and Public Health, 19(9), p.5143.
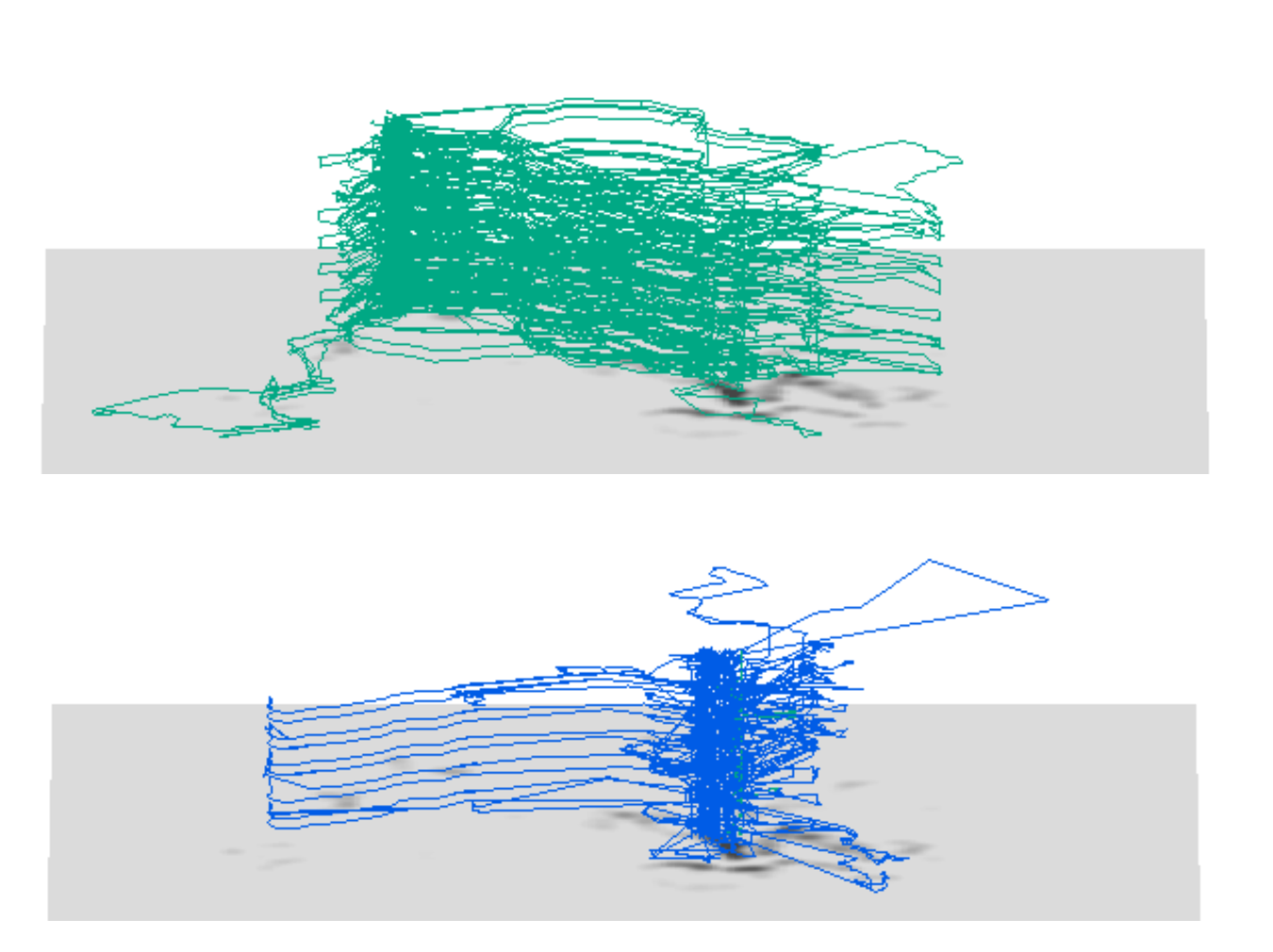
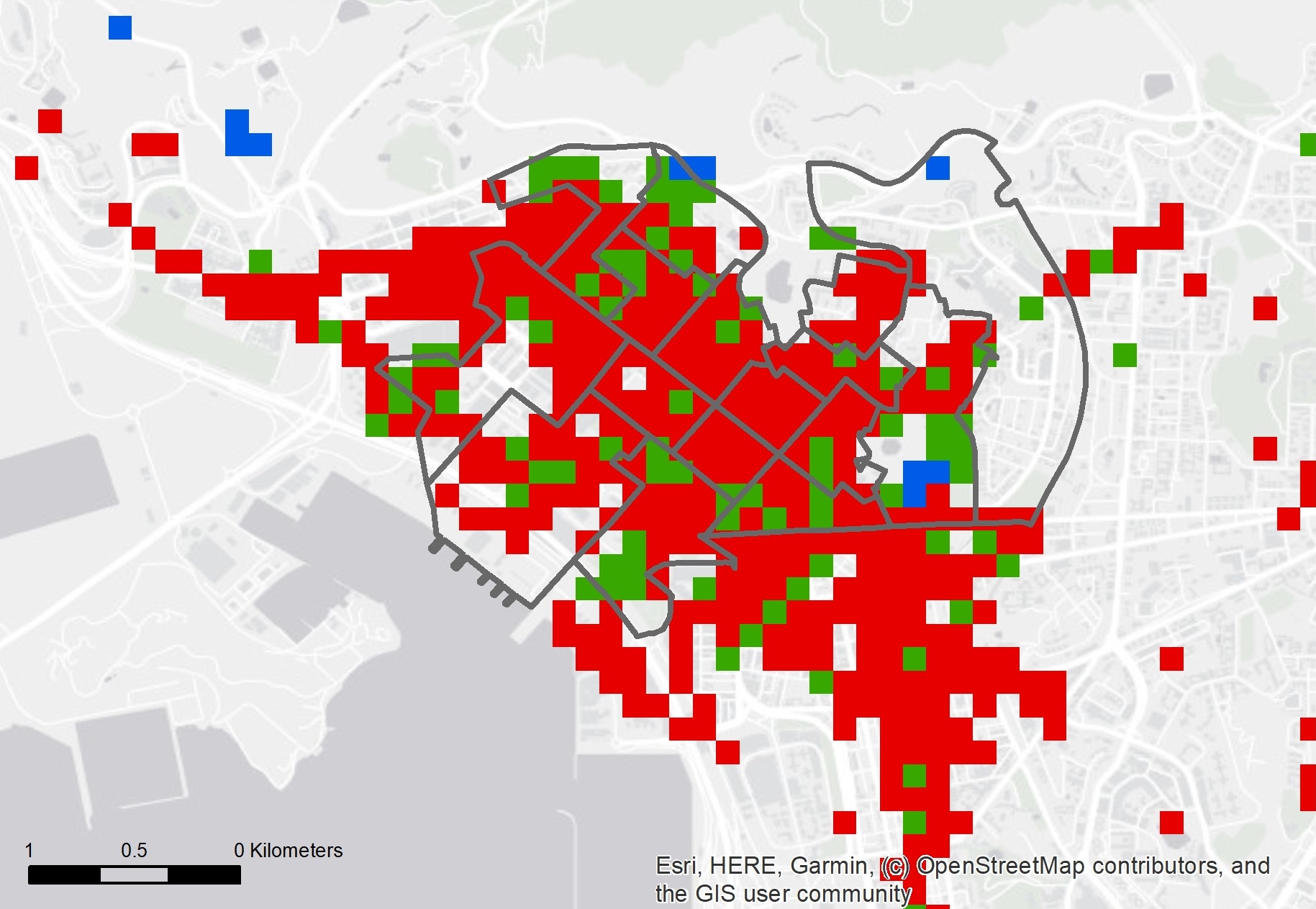
I delve into the dynamics of environmental risks, such as disease transmission risk and air pollution, and explore their potential interactions with human activities and the built environment. Through my studies, I identify regions in Hong Kong with a higher risk of disease (e.g., COVID-19) transmission. By leveraging contact tracing data and a range of geospatial datasets including 3D building data, land cover data, and airborne LiDAR data, I analyze the associated built environment and socioeconomic factors contributing to these risks. Furthermore, using vehicle GPS trajectory data, I investigate the increased risk of vehicle emissions along road networks and assess the impacts of individual vehicle behavior on emission levels.
Kan, Z., Kwan, M.P., Wong, M.S., Huang, J. and Liu, D., 2021. Identifying the space-time patterns of COVID-19 risk and their associations with different built environment features in Hong Kong. Science of the Total Environment, 772, p.145379.
Huang, J., Kwan, M.P. and Kan, Z., 2021. The superspreading places of COVID-19 and the associated built-environment and socio-demographic features: A study using a spatial network framework and individual-level activity data. Health & Place, 72, p.102694.
Kan, Z., Wong, M.S. and Zhu, R., 2020. Understanding space-time patterns of vehicular emission flows in urban areas using geospatial technique. Computers, Environment and Urban Systems, 79, p.101399.
Kan, Z., Tang, L., Kwan, M.P., Ren, C., Liu, D., Pei, T., Liu, Y., Deng, M. and Li, Q., 2018. Fine-grained analysis on fuel-consumption and emission from vehicles trace. Journal of cleaner production, 203, pp.340-352.
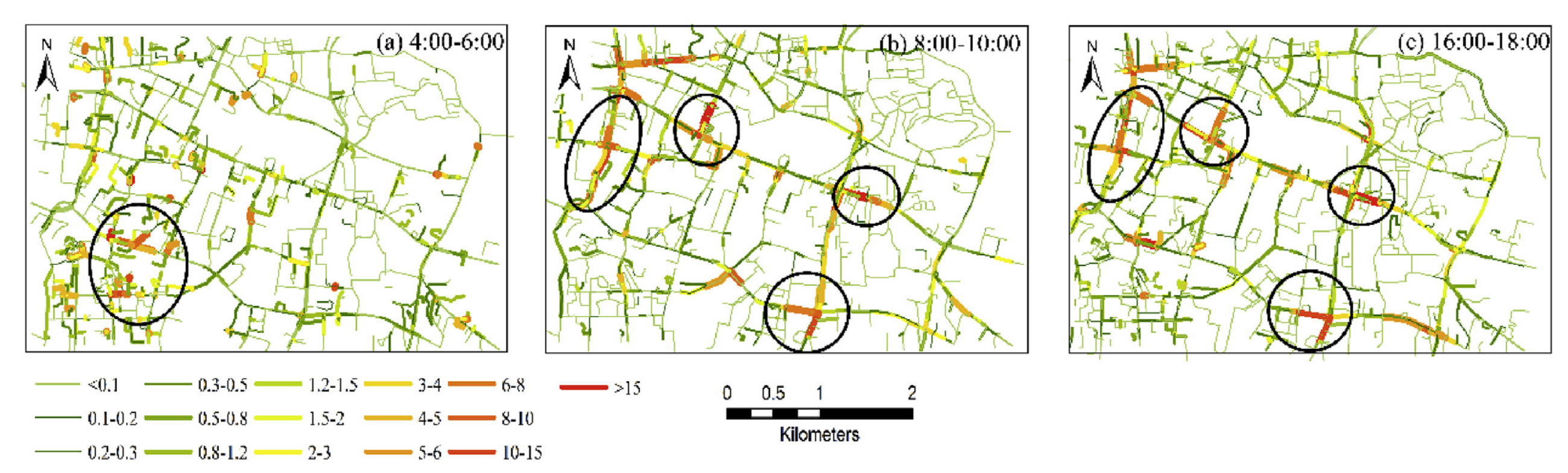
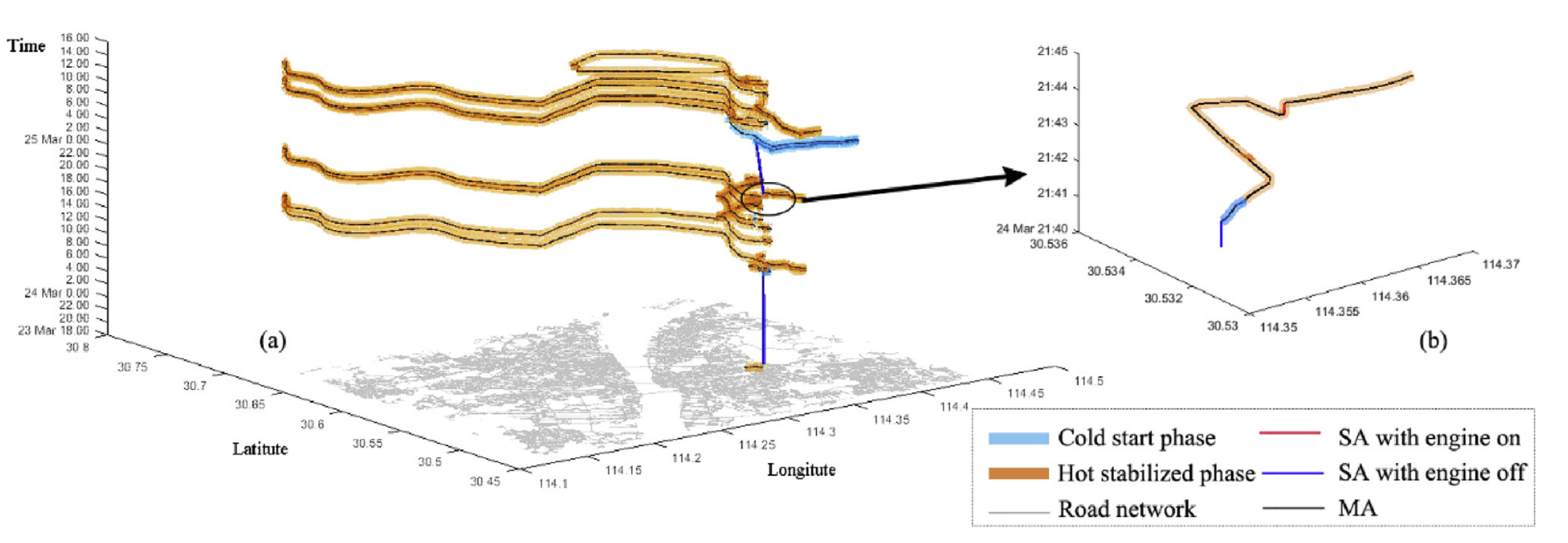